Predictive policy: the new frontier in government
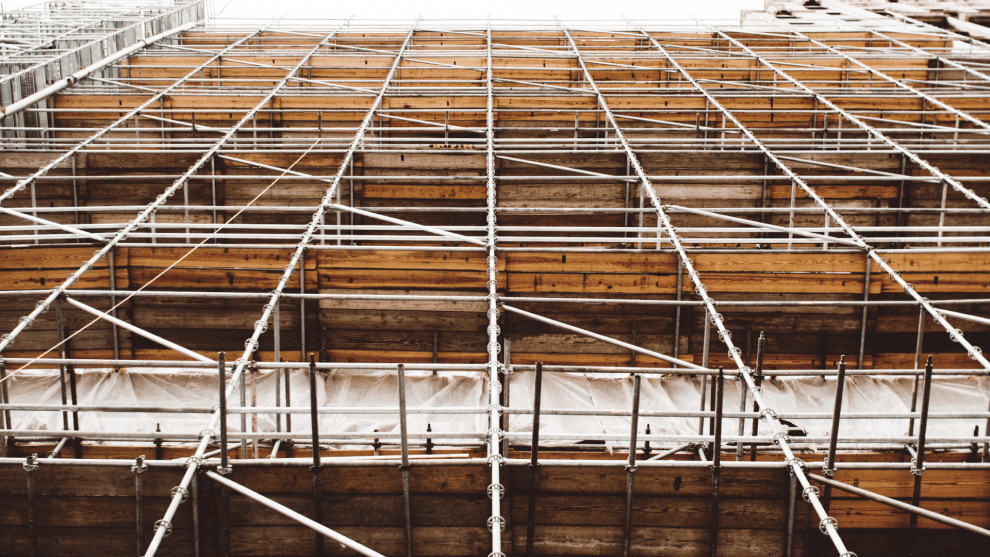
It is a reality that businesses are increasingly using predictive analytics to achieve their outcomes – in marketing, pricing strategies and improving operations. Likewise, governments are also turning to data and AI to develop more timely, targeted and relevant policy for citizens. The increase in data-driven decision making and predictive analytics is palpable.
We increasingly feel the touch of predictive analytics in our daily lives, whether it is a text message from our credit card provider asking if a recent purchase is legitimate, to choosing the next show to binge-watch from the recommendations Netflix is providing or Google Maps showing us the quickest predicted route home.
There is a growing expectation that government policy is not only evidence-based but that it is based on contemporary evidence development including big data, predictive and prescriptive analytics. Thus, we are beginning to enter an age of predictive policy: using predictive and prescriptive analytics to anticipate problems or issues and formulate policy and programs that are preventative rather than reactive.
Predictive policy use in government
- Fraud prevention: tax and social services policy
- Health care: risk scoring in chronic disease prevention and reduction of hospital re-admissions
- Weather: in emergency management responses to natural disasters, flight safety and water flow management.
Similarly, you only need to scratch the surface to find areas of government policy and programs which are yet to effectively engage with their evidence-base and data, let alone predictive analytics.
For those that are interested in delivering effective, efficient and practical policy how do you make the leap to engaging in predictive policy?
It starts with these five things:
- Problem definition: Knowing your policy or program area well allows you to understand what the opportunities and challenges are. Clear definition of the issue is essential to targeting a response. The problem could be one that you experience on a day to day basis or a problem you observe others having. Getting to the heart of the problem is the first step.
- Data: You need enough data, relevant data and high-quality data. Whether your problem is gnarly or completely straightforward you won’t get far in predictive analytics without data. Getting to know your own data, seeking out other’s relevant data and plugging gaps and increasing quality are often foundational steps on the path to useful insights.
- Accessible analytics tools: There are loads of analytics tools out on the market now and most organisations have one (or more) bashing around somewhere BUT in most cases widespread access is limited. To be more data-driven we need egalitarian access to analytics tools to explore the data available to us.
- Culture: Evidence-based practice is really a no-brainer in terms of what we should be aiming for, but in practice, other things get in the way. By fostering an evidence-based culture we increase both the demand and supply of evidence and evidence-based policy.
- Workforce capabilities: Organisations rarely have a holistic understanding of the exiting skills and capabilities within the workforce or of the future skills and capabilities required to deliver on your predictive analytics and predictive policy ambitions. There are specific skill sets and capabilities that need to be fostered in order to deliver on predictive policy. If you don’t have these, not all is lost: consider how to future-proof your workforce or outsource your needs.
Grosvenor Public Sector Advisory: We exist to improve public sector performance. We provide evidence-based advice and solutions, coupled with practical ways to achieve change. Contact our expert and authentic team today.